KIS-DPD: A Simple Framework for Developing Data Products
- Alessandro Nardinelli
- Nov 19, 2023
- 6 min read
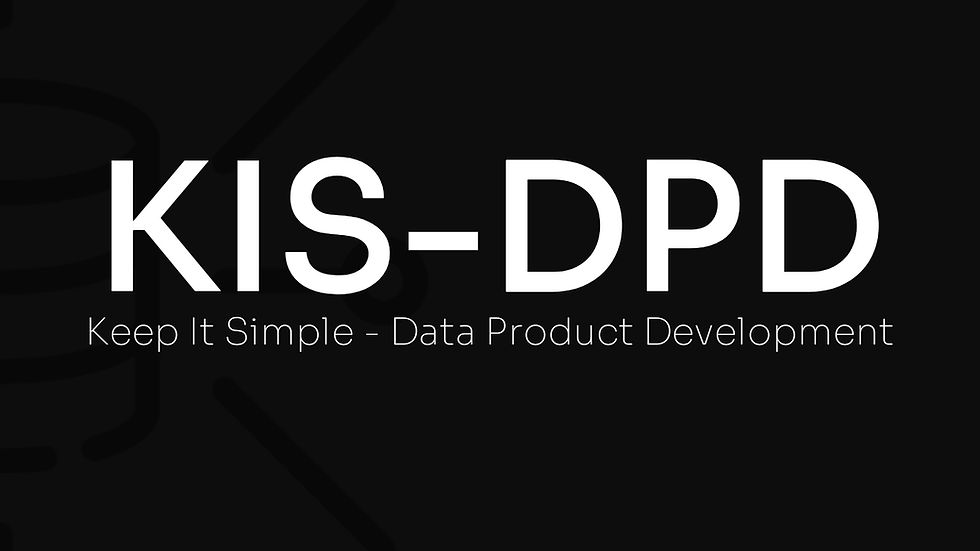
Through my journey of developing mainly products that suggest ways of organizing, recommending, or classifying things, I've learned that they all can fit into specific product development buckets. By using the correct one, we can shift from the cost-intensive, hard-to-explain, and "it's not solving my problem" products to the clear data money-makers that every company wants.
Reading time: ~6 Minutes
Key Words: Data Product Management, Framework, Data Management, Insights, Product Management, Data Team Management
Overview
I am not a big fan of frameworks. They require you to step into a pre-made recipe that does not always work, and while you still follow all the guidelines and take measurements to check if you constantly are on track, it still falls into traps and issues the initial design of the framework did not contemplate. The thing doesn't go well and you keep blaming yourself for it.
The good news is that it is not your fault.
While implementing frameworks can help, it is important to notice that every company, product, and team is different. My reality on writing this is definitively different than yours, in a scenario that only you know.
This is why I begin this article by making two big initial statements:
Use a framework as a baseline for development, not as a definitive true north. It helps as you sail through the bay, but you will have to correct your path and repair your ship on the ocean by yourself. This is where the beauty of the good leader lies, and it should be where you can prove yourself.
This is a framework that is constantly changing, and I need your help to design something that reaches the end goal. This is why this work is Open Source, shared under the Attribution-ShareAlike 4.0 International. I've selected a license that is pretty easy to understand and also enables people to work along without hidden footnotes, but please read here what you can and cannot do with this work. You can find all the open-source files in this Miro template link.
Connecting Core OKRs
Data products are key initiatives to help the company grow into a more profitable and organized structure. A study from McKinsey shows that many core executives still have a mind fixed on the reporting side of data while having a hard time understanding how advanced data roles and products could impact their business positively.[1]
Being a Data Product Manager involves a great capacity to understand the core OKRs the company has to help leverage those initiatives clearly with data products. This is key because it showcases how the area is connected to these main goals of the team and also the high management. The lack of doing so can result in those leaders not having super clear what their data team can do, and so it maintains that feeling of "please just bring me the report I want for the board meeting".
To connect is pretty simple, but you should pay close attention to not deviate from the main objectives once your mind flows into a more technical data state, which is pretty easy for data heads.
The OKR Canvas
To help with this task, the Canvas below tries to make a fluent transition between company OKRs and how the data team should tackle the products.
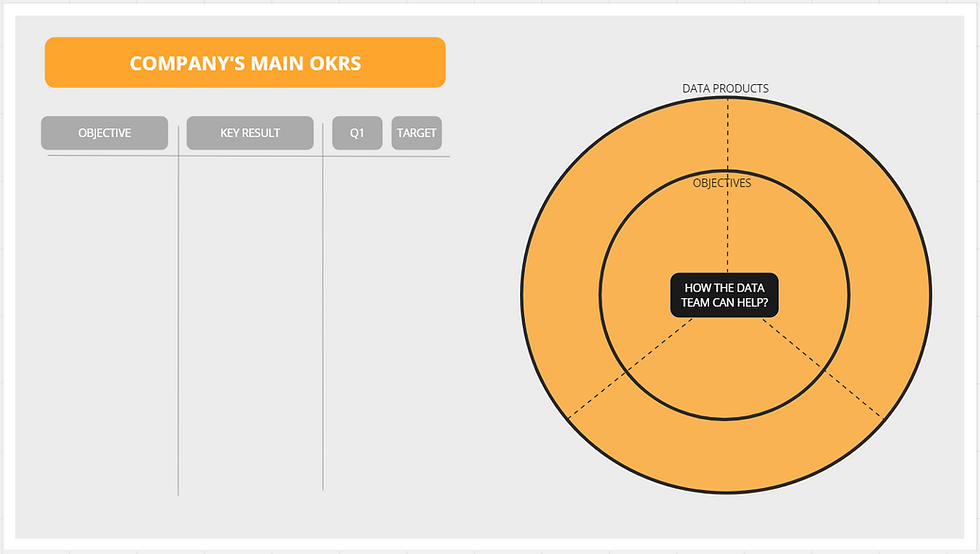
Start by focusing on describing the main company goals. You can do this by simply talking to the managers yourself or with your boss. Ideally, all companies should have this pretty clear when they set their goals for the year, semester, or quarter.
In the example below, I painted generic goals just so you know how the exercise goes along.
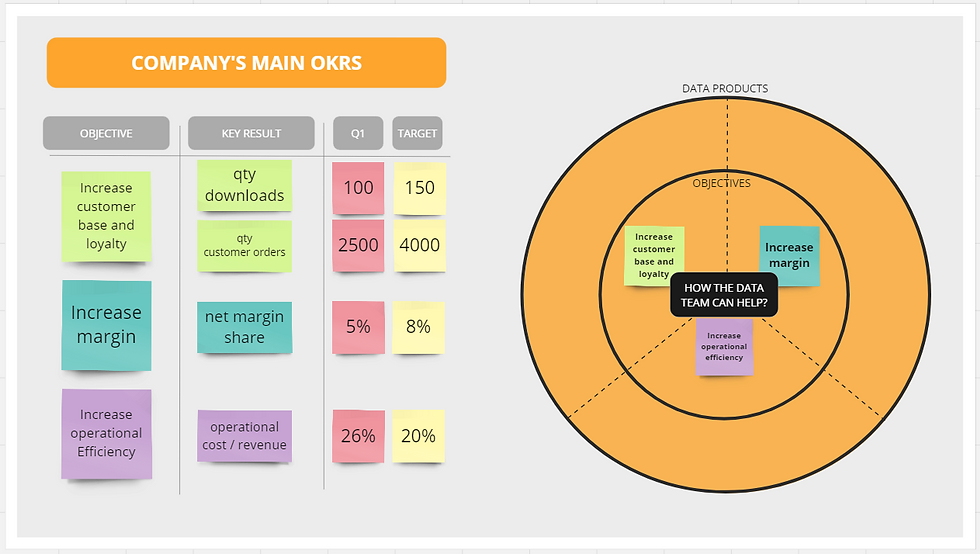
Once you have the goals set on the table(left), just copy them to the inner circle. The main idea is to directly connect the data products with the core objectives into one slide only.
Finally, fill the outer circle with products that make a clear connection. If you can identify products that fulfill more than one objective, you will find what I call "star products". Those are initiatives that tackle two things at once and should be prioritized since they can speed things up a lot, and the effort will really make things better.

By making it clear what the company's OKRs are and how your data products connect, you, the team, and the main stakeholders can understand simply without too many questions with only one slide. You might be prompted to explain why those products are the real choice, but it should be fairly easy to understand from this one central idea.
Identifying Initial Action Classes
This framework separates all the data products and initiatives into two main categories: Structural and Insights. You should be able to categorize most of the things you do with data on one of these buckets:
Inside the structural bucket, you place actions that are necessary to maintain security, governance, data flow (ETLs and ELTs), as well as infrastructure and FinOps. Here you should focus your roadmap on keeping things tight and running smoothly, as well as not costing too much.
Inside the Insights bucket, you place the initiatives that are directly connected to business actions, such as models, overall analytics, and customer-centric products.
If you already have data processes and products, it should be somewhat easy to classify them there. If you don't, remember to connect them to what is going to most impact the business, so you can move forward in communicating it clearly once you start to develop them. A list should be enough. Remember that the idea is not to overcomplicate things.
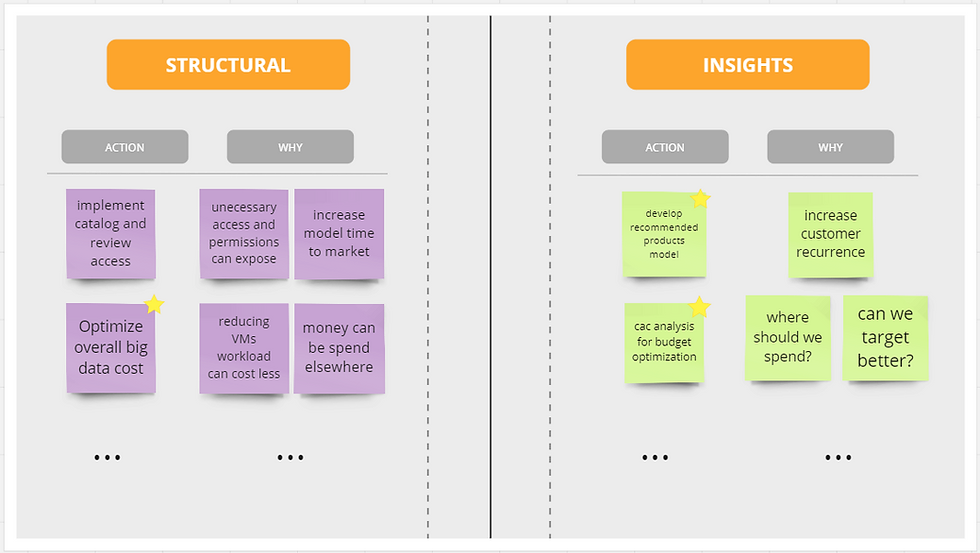
The dotted lines in the middle are used for things that somehow can be on both sides, such as analytics engineering for example. Since those are more advanced, and if you do have them in your portfolio, feel free to create a transition category, but I tend to include them on the Insights bucket since it will add tangible value to the core business in the end.
Please remember to connect the actions with the previous step.
It is really important to have a clear link between the actions and products the team will develop with the company's main OKRs because it gives them a clear purpose and sets a tangible path of product development so that the company's main objectives are achieved.
Roadmap Management for the Data Product Management
Your roadmap should be designed in such a way that you can communicate the connection to your company's main OKRs without too many questions around it. It should, as this framework is, simple enough to understand at first sight. I also do not tend to get creative here, since it's made more for your communication as a facilitator with both the team and the stakeholders showcasing difficulties, deadlines, and owners.
The following model should work fine for this. Remember to keep the stars on as you walk down the path to remind yourself and all the stakeholders that those are the ones that everybody should be committed enough to make happen. If you want to keep a more detailed track in a tool that you already use, you're welcome to do so (and it's highly recommended since these products and initiatives can be broken down for better tracking) but stick to the easy explanations for non-tech managers, and keep the geek-speak to your team.
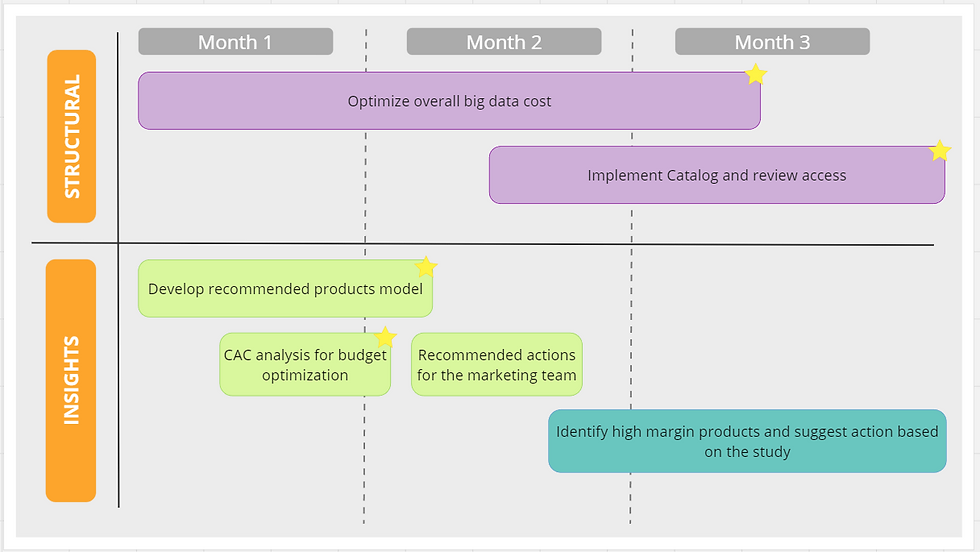
You can also add flags and other icons/colors to increase the power of the storytelling about the roadmap, but as an example, I'm going to keep it simple. If you want to better understand how to create and build a roadmap and plan of action, my suggestion is to read this article.
The KIS-DPD Product Sheet
While understanding a digital product is easy enough to do when they have buttons on a screen, some data products require a little bit more clarification since most of the time they are not things you can see or click. To do that, create a single slide for each product trying to describe the main objective, and the milestones to achieve it.
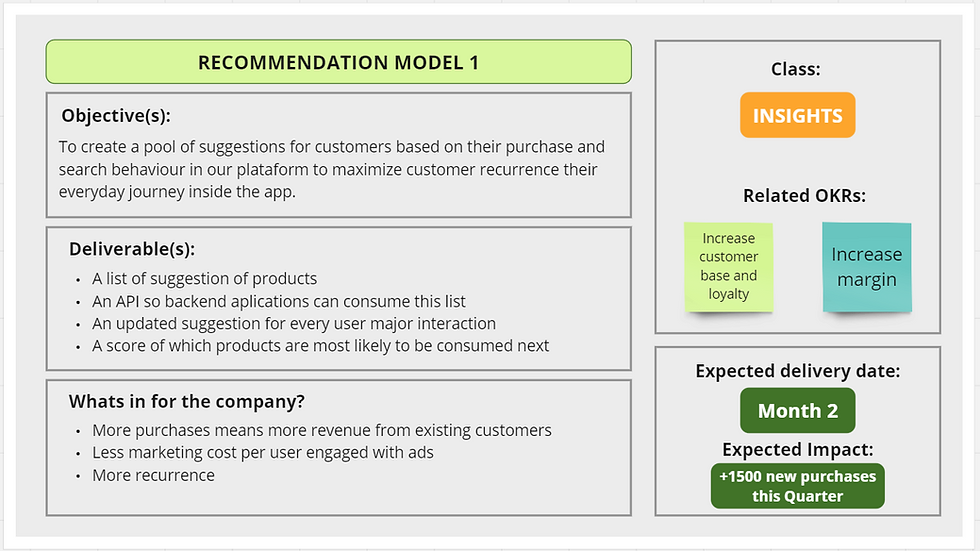
This is a powerful Canva because it is easy for every stakeholder of the project to understand, ranging from the nerdy data scientist to the "I don't have time" executive. Forwarding this slide in an email of the formal invitation to the weekly roadmap showcase could clarify and speed things up a lot. Remember that the discussions around data products must not have a technical hamburger at the table, otherwise, people will quickly lose focus on the discussion.
Wrapping Up
With the fast technical advances in data products, it is hard to keep up the pace, mainly if you also have to be on the business side. Senior managers want to quickly understand the impacts those products can bring, but prefer also to "leave it with the experts", just to come two months after asking for the new GPT oracle. As data and product professionals, our goal is to identify big opportunities while keeping our feet on the ground. Your ability to communicate effectively will be a great opportunity to showcase the power these products can make but also can become a geek-talk fast, disengaging, or losing those money-makers fast.
During my time perfecting this framework in countless meetings, team alignments, and board presentations, I realized that the simpler, the better. It will help you with less thinking about how to explain what you do to your team, managers, and (why not), grandma.
[1] Díaz, A., Rowshankish, K., Saleh, T. (2018). Why Data Culture Matters. A McKinsey Quarterly Report
KIS-DPD © 2023 by Alessandro Nardinelli is licensed under CC BY-SA 4.0
Comments